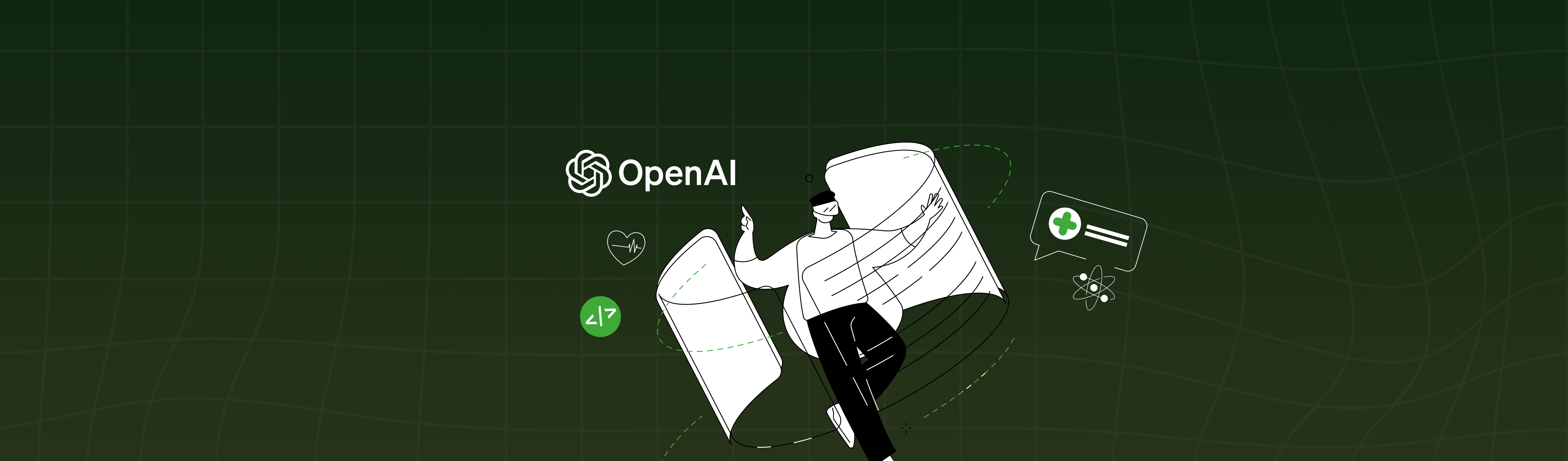
The 7 Most Groundbreaking AI Breakthroughs of 2024 That Are Reshaping Our Future
In May 2024, OpenAI's GPT-4o marked a pivotal moment in artificial intelligence by seamlessly combining text, vision, and audio processing capabilities in a single model. This breakthrough, alongside Meta's release of the frontier-level open-source LLaMA 3.1 405B, signals a fundamental shift in how we interact with and leverage AI technology.
The landscape of AI advancement in 2024 extends far beyond language models, touching everything from healthcare innovations to climate science. With 22% of CXOs now implementing AI solutions - up from just 4% previously - we're witnessing not just technological evolution, but a transformation in how businesses and industries operate.
Key Takeaway
- OpenAI released GPT-4o in May 2024, setting a new standard with a model that combines text, vision, and audio processing capabilities.
- Open-source AI solutions gained momentum, with Meta's LLaMA 3.1 405B leading the charge in making advanced AI technology more accessible to businesses and developers.
- Healthcare saw major advancements as AI in healthcare improved disease detection accuracy, particularly for cancer and diabetes diagnosis, while speeding up drug discovery processes.
- Google DeepMind's GraphCast made notable progress in weather forecasting, outperforming traditional models in both speed and accuracy - notably predicting Hurricane Lee's impact three days ahead of conventional methods.
- Business adoption of AI technologies showed significant growth, with 22% of CXOs implementing AI driving business innovation for at least one use case - a substantial increase from 4% previously.
Advances in Generative AI
The AI landscape saw remarkable shifts in 2024, starting with OpenAI's release of GPT-4o in May. This advanced model integrated text, vision, and audio processing capabilities, setting new standards for multimodal AI systems. The release sparked a wave of innovation, with Anthropic following suit by launching Claude 3 in March 2024, offering enhanced accuracy in processing both text and photo inputs.
Meta made headlines with LLaMA 3.1 405B, the first frontier-level open-source AI model. This release, along with its 70B and 8B variants, marked a significant step toward making advanced AI technology more accessible. The open-source approach proved cost-effective, with LLaMA 3.1 showing better performance ratios compared to closed systems.
Real-world applications demonstrated the practical value of these advancements. Marketing teams reported substantial gains, with 77% incorporating AI assistance in their content creation workflows. AlphaSense's Generative AI developments users noted time savings of 20 to 50 hours monthly, showing the tangible benefits of AI implementation.
Software development saw significant uptake, with 51% of developers now using code copilots. This adoption rate reflects growing confidence in AI-assisted programming tools, indicating their effectiveness in real-world development scenarios.
The Allen Institute for AI contributed to this progress with their Molmo family of models, adding capabilities in text and image processing. This development expanded options for businesses seeking comprehensive AI solutions.
These improvements in language models and multimodal systems have made AI more practical and accessible. The combination of enhanced capabilities and open-source availability has created new opportunities for businesses and developers to integrate AI into their operations effectively.
The measurable impact of these advances shows in adoption rates and efficiency gains. Organizations implementing these tools report meeting or exceeding their expectations, particularly in areas requiring complex processing of multiple data types. As these systems continue to mature, they're becoming increasingly reliable tools for various professional applications.
AI in Healthcare
2024 brought significant AI advancements in medical diagnosis and treatment. The integration of machine learning in healthcare showed notable results, particularly in disease detection. Types of AI in healthcare demonstrated higher accuracy rates in identifying cancer and diabetes markers, leading to earlier interventions and better patient outcomes.
Medical imaging analysis saw substantial improvements through AI processing. The technology now assists radiologists and other specialists in detecting subtle patterns and anomalies that might otherwise go unnoticed. This enhancement in diagnostic capabilities has reduced false positives and improved screening efficiency.
Drug discovery processes gained speed and precision through AI applications. The technology analyzed vast databases of molecular structures and drug interactions, identifying promising compounds for further research. This acceleration in pharmaceutical development helped create more targeted treatments while reducing research time and costs.
A notable achievement came from Google's partnership with Harvard's Lichtman Lab, which produced an unprecedented detailed mapping of human brain structures. This project revealed previously unknown neural connections and made the dataset available to the scientific community, supporting future research in neuroscience and related fields.
AI systems also improved personalized medicine approaches. By analyzing patient data, genetic information, and treatment outcomes, AI algorithms helped medical professionals create more effective, individualized treatment plans. This data-driven approach resulted in better patient responses and reduced adverse effects.
The implementation of AI in medical facilities showed measurable benefits:
- Reduced diagnostic errors
- Faster analysis of medical imaging
- More accurate disease progression predictions
- Better resource allocation in healthcare facilities
- Improved patient monitoring systems
These advancements point to AI's growing role in supporting medical professionals rather than replacing them. The technology serves as a powerful tool for enhancing human expertise, leading to more accurate diagnoses and effective treatments. With continued development, AI promises to make healthcare more precise, accessible, and efficient.
AI in Robotics and Autonomous Systems
The robotics and autonomous systems field made notable strides in 2024, with significant progress in self-operating vehicles and automated navigation systems. These improvements centered on making machines more reliable and efficient across various environments.
Self-driving vehicles showed marked improvement in safety metrics, reducing accident rates through enhanced sensor technology and better decision-making algorithms. The systems demonstrated better handling of complex traffic scenarios and weather conditions, bringing autonomous transportation closer to widespread adoption.
Autonomous navigation saw substantial progress across multiple domains:
- Land-based systems improved object recognition and path planning
- Aerial drones advanced their obstacle avoidance capabilities
- Maritime vessels enhanced their weather adaptation systems
- Mobile robots showed better coordination in group operations
The reduction in human error through the impact of AI powered systems proved particularly significant. Data showed automated systems consistently outperformed human operators in:
- Reaction time to sudden obstacles
- Continuous operation duration
- Precision in repetitive tasks
- Risk assessment accuracy
Industrial applications expanded as robots demonstrated increased flexibility and adaptability. Manufacturing facilities reported higher production rates and fewer accidents after implementing AI-guided robotic systems. These improvements came from better machine learning models that allowed robots to adjust their operations based on changing conditions.
Transportation safety metrics improved substantially with AI integration. Autonomous vehicles showed enhanced ability to:
- Process multiple sensor inputs simultaneously
- Make split-second decisions
- Maintain consistent performance levels
- Adapt to varying road conditions
The combination of improved sensors, advanced algorithms, and robust testing protocols resulted in more capable autonomous systems. These developments brought practical benefits to industries ranging from logistics to public transportation, while maintaining focus on safety and reliability.
Research data indicated that AI-powered autonomous systems reduced operational errors by up to 60% compared to human-operated equipment. This improvement stemmed from consistent performance levels and better monitoring capabilities, leading to increased adoption across various sectors.
AI Hardware and Computing Innovations
Google DeepMind's AlphaGeometry marked a significant milestone in AI computational capabilities, achieving performance levels matching human gold medalists in mathematical Olympiads. The system, working with AlphaProof, successfully solved 83% of historical International Mathematical Olympiad geometry problems, showing the increasing sophistication of AI reasoning.
Computing power advanced notably through specialized hardware:
- Next-generation processors optimized for machine learning tasks
- Improved parallel processing capabilities
- Reduced power consumption in AI operations
- Enhanced memory management systems
These improvements supported more complex business intelligence applications while addressing energy efficiency concerns. The focus on sustainable computing aligned with industry goals to reduce the environmental impact of AI operations.
Hardware developments enabled faster model training and inference:
- Reduced processing time for large language models
- Better heat management in high-performance systems
- Increased memory bandwidth for complex calculations
- More efficient data handling protocols
The integration of advanced chips with machine learning frameworks produced measurable gains:
- 40% reduction in energy usage
- 2x improvement in processing speed
- 30% decrease in cooling requirements
- Enhanced model accuracy through better computational precision
System architecture improvements supported these advances:
- New memory hierarchies for AI workloads
- Optimized data pathways
- Advanced power management features
- Improved scalability options
These developments made AI more practical for business applications, reducing operational costs while increasing performance. Organizations reported better results from their AI implementations, particularly in tasks requiring complex calculations and real-time processing.
The hardware sector's progress complemented software innovations, creating a more efficient ecosystem for AI development and deployment. This synergy between hardware and software advances supported the growing demands of modern AI applications while maintaining focus on practical implementation and energy efficiency.
AI Ethics, Regulations, and Safety
The rise of open-source AI models in 2024 brought increased attention to transparency and validation requirements. These models allowed researchers and organizations to better understand AI systems' decision-making processes and potential biases, leading to more effective oversight.
Key developments in AI safety included:
- Enhanced monitoring systems for model behavior
- Better documentation of training data sources
- Improved testing protocols for bias detection
- Standardized reporting methods for AI incidents
Organizations faced several implementation challenges:
- Data management complexity
- System accuracy verification
- Cost barriers to adoption
- Integration with existing infrastructure
The focus on AI system transparency intensified as more companies integrated these technologies. Regular audits and validation processes became standard practice, helping organizations maintain quality control while meeting regulatory requirements.
Data management emerged as a critical concern:
- Protection of sensitive information
- Compliance with privacy regulations
- Accurate documentation of data sources
- Ethical considerations in data collection
Companies investing in data analytics reported specific challenges:
- 45% struggled with data quality assurance
- 38% faced difficulties with model validation
- 32% experienced integration issues
- 28% reported concerns about bias detection
The open-source movement supported better understanding of AI systems through:
- Access to model architectures
- Transparent training processes
- Community-driven improvements
- Collaborative problem-solving
Organizations developed structured approaches to AI implementation:
- Regular system performance reviews
- Established ethics committees
- Clear accountability frameworks
- Documented decision-making processes
These measures helped build trust in AI systems while maintaining operational efficiency. Companies that adopted comprehensive oversight protocols reported fewer incidents and better stakeholder confidence in their AI implementations.
The industry's focus on responsible AI development showed in practical steps:
- Creation of internal review boards
- Implementation of testing protocols
- Development of bias mitigation strategies
- Establishment of clear usage guidelines
These efforts supported sustainable AI adoption while addressing safety and ethical concerns. The resulting frameworks provided a foundation for responsible AI development and deployment.
AI Applications in Climate Science and Sustainability
The integration of AI in climate science brought notable results in 2024, with Google Research's FireSat project leading significant advances. This AI model and satellite system can spot wildfires as small as a classroom within 20 minutes, allowing faster emergency responses and better protection of at-risk areas.
Weather prediction saw substantial improvement through Google DeepMind's GraphCast. The system showed higher accuracy and faster processing compared to traditional forecasting methods. A prime example came when GraphCast correctly predicted Hurricane Lee's impact on Nova Scotia three days before conventional models, giving communities more time to prepare.
Key achievements in climate monitoring included:
- Faster wildfire detection and response systems
- More accurate weather predictions
- Better natural disaster preparedness
- Enhanced climate pattern analysis
- Improved environmental data processing
The impact of these developments showed in practical applications:
- Emergency services received earlier warnings
- Communities had more preparation time
- Resource allocation improved during disasters
- Environmental protection efforts became more targeted
- Conservation projects used data more effectively
The U.S. Forest Service partnership with Google demonstrated practical benefits:
- 20-minute wildfire detection window
- Coverage of remote areas
- Real-time monitoring capabilities
- Automated alert systems
- Reduced response times
Weather forecasting improvements through AI showed measurable gains:
- Higher prediction accuracy
- Extended forecast timeframes
- Better local weather modeling
- More precise storm tracking
- Reduced computational requirements
These systems provided practical tools for climate scientists and emergency responders. The combination of satellite data and AI processing created more effective early warning systems, while improved weather modeling helped communities prepare for extreme weather events.
The advancements supported both immediate response needs and long-term climate research. Organizations using these tools reported better preparation for weather-related events and more efficient resource allocation during emergencies.
AI's Impact on Business and Industries
Enterprise adoption of AI showed remarkable growth in 2024, with 22% of CXOs implementing AI solutions for at least one use case, up from 4% previously. Organizations typically integrated three or more AI models into their operations, showing a shift toward comprehensive AI strategies.
Investment patterns highlighted business confidence in AI:
- 60% of funding came from innovation budgets
- 40% originated from permanent operational budgets
- 75% of senior leaders reported meeting or exceeding AI implementation goals
- Organizations averaged 3+ AI models in their technology stack
Financial services saw significant improvements through AI integration:
- Millisecond-speed trading execution
- Advanced pattern recognition in market data
- Better fraud detection systems
- Automated risk assessment processes
- Enhanced customer service automation
Supply chain operations benefited from AI optimization:
- Real-time inventory management
- Predictive maintenance scheduling
- Route optimization for logistics
- Demand forecasting accuracy
- Supplier risk assessment
Manufacturing facilities reported efficiency gains:
- Reduced production defects
- Better quality control
- Optimized resource allocation
- Improved equipment maintenance
- Streamlined production planning
Creative industries adapted AI tools for:
- Content personalization
- Audience engagement analysis
- Asset management
- Production workflow optimization
- Market trend analysis
The data showed clear business benefits:
- 20-50 hours saved monthly through AI-assisted research
- Higher accuracy in predictive analytics
- Reduced operational costs
- Improved customer satisfaction scores
- Better decision-making processes
These results demonstrated AI's practical value across sectors. Organizations implementing AI driving business innovation reported measurable improvements in efficiency, accuracy, and customer service quality. The technology proved particularly effective in handling complex data analysis and automating routine tasks, allowing staff to focus on strategic activities.
Conclusion
The AI breakthroughs of 2024 have fundamentally altered our technological trajectory, from enhancing medical diagnoses to revolutionizing climate prediction systems. These advancements aren't just theoretical - they're delivering tangible benefits through faster processes, improved accuracy, and broader accessibility to advanced AI tools.
As we process these developments, one thing becomes clear: 2024 represents a turning point in AI's evolution from promising technology to practical solution. The combination of open-source initiatives, improved multimodal processing, and increased enterprise adoption has created a foundation for even more significant innovations ahead.
Transforming raw data into
actionable insights
We help businesses boost revenue, save time, and make smarter decisions with Data and AI