
How Bad Data is Costing Companies Millions and How to Fix It
Bad data is a silent killer.
It creeps into your systems unnoticed, quietly draining your business resources.
Inaccurate or inconsistent data undermines trust, efficiency, and profits.
Data should drive your decisions, but when it's unreliable, it can lead to costly mistakes and missed opportunities.
The solution lies in finding and fixing these issues before they spiral out of control.
Key Takeaways
- Bad data silently impacts your business, leading to operational inefficiencies, poor decision-making, and lost profits.
- Inaccurate data compromises trust in systems, causing delays and hesitation in acting on insights.
- AI-powered automation and data validation tools can help cleanse and maintain the integrity of your data in real-time.
- Self-healing data pipelines use AI to detect and correct data issues automatically, ensuring smooth data flow.
- Real-time monitoring and data observability platforms help catch anomalies before they escalate into larger problems.
- Implementing data governance frameworks ensures consistent data handling and reduces the risk of compliance issues.
The Hidden Costs of Bad Data
Bad data isn't always obvious, but its impact can be felt across every department. It silently creeps into your business processes, causing issues that range from misinformed decisions to operational inefficiencies. These hidden costs of bad data often go unnoticed until they have compounded into major problems.
Let's break down how bad data affects different aspects of your organization:
1. Decision-Making is Compromised
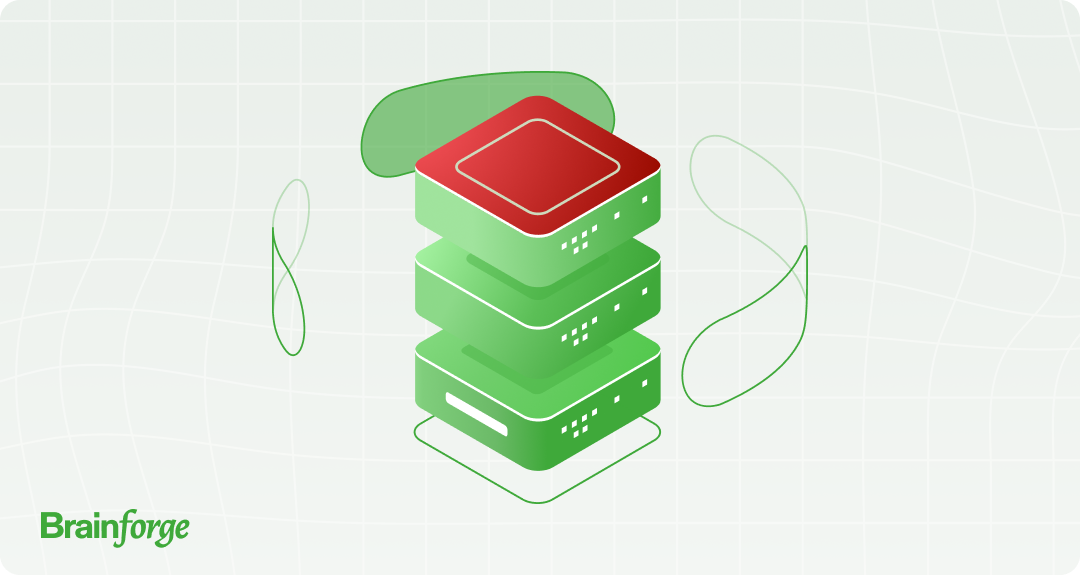
When teams work with inaccurate data, the decisions they make are unreliable. Whether it's forecasting future trends, analyzing customer behavior, or adjusting financial strategies, decisions based on bad data can lead to missed opportunities and costly errors.
IBM found that poor data quality costs companies $3.1 trillion annually in the United States alone. This staggering number reflects how widespread data issues can directly affect decision-making at every level, from strategic to operational decisions
In modern data pipelines, errors are most commonly introduced during data extraction or transformation. Using ETL processes, such as those implemented in DBT, you can clean and validate data at each step of its journey. DBT helps automate transformations and ensures that data conforms to predefined quality standards before it's used in decision-making processes.
2. Loss of Trust in Data Systems

One of the most critical yet intangible effects of bad data is the erosion of trust. When teams discover that they've made decisions based on inaccurate data, trust in the entire data system can collapse. Teams may hesitate to act on insights, causing delays and reducing operational efficiency.
Maintaining trust in your data requires implementing robust data validation processes. Automated tools such as data observability platforms and real-time monitoring solutions are essential for continuously tracking data health. Platforms like Rill Data provide visibility into data pipelines, allowing teams to monitor changes, track anomalies, and catch data issues before they impact reports.
3. Increased Operational Costs
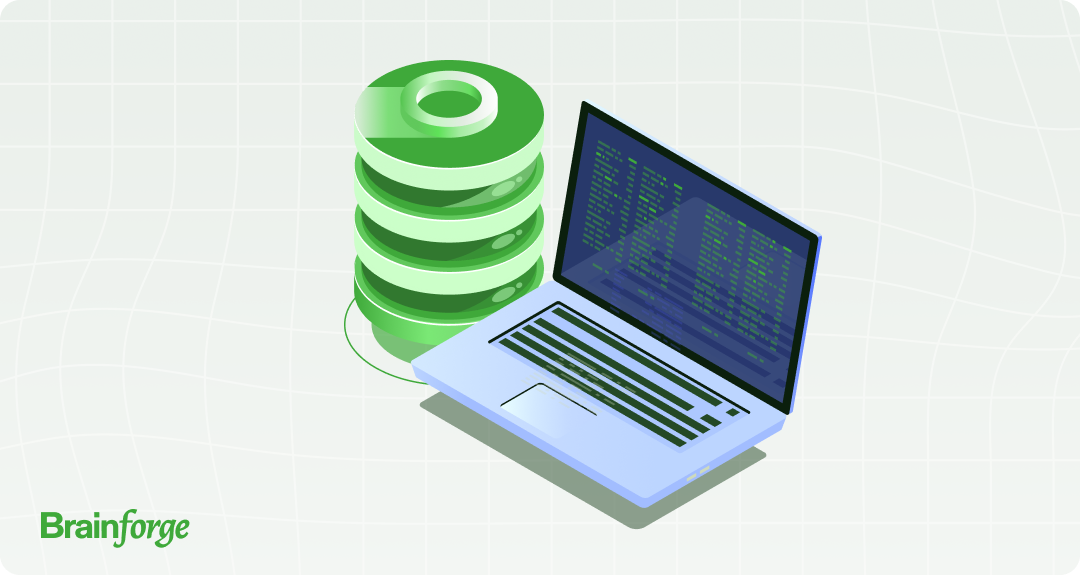
Bad data often leads to operational inefficiencies that are difficult to quantify but have real financial implications. Inaccurate inventory data might result in overstocking or understocking products, and errors in supply chain data could lead to delays and higher costs.
Operational data can be validated and optimized using automated monitoring systems. These systems can track key operational metrics in real-time and identify inconsistencies before they escalate. For instance, using real-time anomaly detection algorithms, operational leaders can set up alerts for when data falls outside acceptable parameters.
4. Reputational Damage
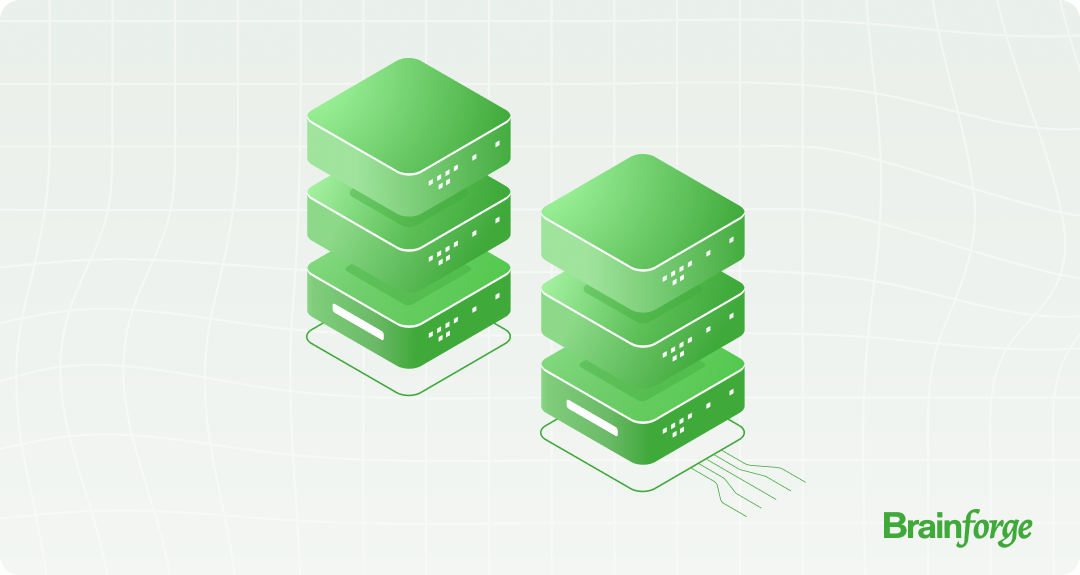
When bad data is used to inform customer-facing processes, it can lead to damaging errors. For example, sending out incorrect invoices, miscommunicating with customers, or delivering the wrong products can tarnish a company's reputation, leading to lost trust and business.
Data governance frameworks ensure that data entering customer-facing systems is validated and accurate. By establishing data quality rules and automated governance tools, companies can enforce standards that prevent bad data from reaching customer touchpoints.
5. Impact on Compliance and Legal Risk

Bad data can also expose your business to legal and compliance risks. In industries like finance or healthcare, inaccurate data can result in non-compliance with regulatory standards, leading to fines and legal challenges.
Implementing a comprehensive data governance strategy ensures that data handling complies with regulations. By automating data lineage tracking and audit trails, businesses can trace any modifications or transformations that data undergoes, making it easier to comply with industry standards and provide evidence during audits.
The Real Cost of Poor Data Quality
Bad data isn't just an inconvenience—it directly impacts your company's financial health. From misleading insights to wasted resources, poor data quality quietly drains profits across the board.
1. Misleading Analytics and Forecasts
When data is inaccurate or incomplete, it skews analytics and leads to poor decision-making. This can cause companies to overestimate demand, under-budget expenses, or misalign operational goals. For example, inaccurate forecast can lead to overproduction resulting in excess inventory and wasted resources. Data transformation tools, such as DBT, help automate the process of cleaning and validating data, ensuring that only accurate information is used in critical business reports.
2. Wasted Marketing Spend
Marketing heavily depends on accurate customer data to reach the right audience. When customer profiles are incomplete or outdated, marketing campaigns become less effective, resulting in a wasted budget. For instance, campaigns might target incorrect demographics or include duplicate contacts. Regular audits and automated data validation processes help ensure customer databases are accurate, allowing companies to maximize ROI from their marketing efforts. With automated data pipelines, customer data is continuously cleaned and updated in real-time, ensuring campaigns hit their mark.
3. Operational Inefficiencies
Bad data often leads to inefficiencies in day-to-day operations. Inaccurate inventory data, for example, can cause overstocking or stockouts, both of which lead to lost revenue. Real-time data monitoring tools help ensure operational data is constantly checked for accuracy, allowing teams to make quick adjustments. These systems monitor key metrics and issue alerts if inconsistencies arise, ensuring smooth operations and reducing costly mistakes.
4. Lost Sales Opportunities
Sales teams rely on accurate data to close deals and follow up with high-value customers. When that data is incomplete or incorrect, opportunities are missed. Whether it's chasing the wrong leads or following up too late, poor data quality can directly impact revenue growth. Automated data governance systems continuously scan and update sales records, ensuring teams always work with the most up-to-date customer information.
Common Sources of Bad Data and How to Fix Them
Bad data can infiltrate your systems through various points, and understanding the sources is the first step toward fixing it.
1. Human Error
One of the most common sources of bad data is manual input. Typos, missing fields, or inconsistent formatting can all lead to significant inaccuracies. For example, duplicate entries or miscategorized information can corrupt your datasets, leading to unreliable reporting and analysis.
Automating data entry processes with AI-driven data validation tools reduces human error by flagging potential mistakes as they happen. These tools can be integrated into data pipelines to ensure that any inconsistencies are caught and corrected before the data moves downstream.
2. Inconsistent Data Across Multiple Systems
In modern businesses, data often comes from multiple sources—customer databases, financial systems, CRM tools, and more. When these systems don't communicate or use different formats, the data can become inconsistent, leading to discrepancies in reporting and decision-making.
Using ETL (Extract, Transform, Load) processes, businesses can integrate data from multiple systems into a unified format. Tools like DBT enable data transformations that standardize data at every step, ensuring that all sources are aligned before analysis. This allows companies to maintain data consistency across the board, avoiding errors caused by mismatched formats.
3. Data Silos
Data silos occur when departments or systems don't share their data effectively, leading to isolated datasets that aren't synchronized with the rest of the company. This can result in outdated or incomplete data, which limits your ability to get a comprehensive view of your operations or customers.
Breaking down data silos requires implementing centralized data warehouses and cloud-based platforms that integrate data from all departments. Real-time data integration tools can synchronize data across the organization, ensuring that everyone has access to the same, up-to-date information.
4. Legacy Systems
Old, outdated systems that haven't been upgraded often produce or store poor-quality data. These systems might lack the ability to validate, cleanse, or integrate data with newer technologies, leading to inefficiencies and inaccurate data handling.
Migrating from legacy systems to cloud-based data platforms allows companies to leverage modern data management tools. Platforms like RillData can replace outdated infrastructure with scalable, real-time solutions that provide faster access to clean data. Additionally, AI-driven data migration tools can help automate the process of transferring data, ensuring accuracy throughout the transition.
Now that we’ve identified the sources of bad data, let's explore how to fix these issues and ensure long-term data quality.
Fixing Bad Data—Strategies for Long-Term Data Quality
Fixing bad data isn't a one-time effort—it requires ongoing processes and systems to ensure long-term data quality.
1. Building Automated Data Pipelines for Consistency
At the core of long-term data quality is the ability to standardize and validate data across systems. Automated data pipelines are essential for handling large, complex datasets that require transformation, validation, and monitoring as they move through various platforms. These pipelines can be built using tools like Apache Airflow or dbt, and they ensure that data is transformed and validated consistently before it's loaded into your data warehouse or lake. For example, dbt allows you to define data quality tests within your transformation scripts, ensuring that data conforms to predefined rules and constraints.
2. AI-Driven Data Cleansing for Continuous Quality
Traditional data cleansing methods are reactive and often manual, which leaves room for error. AI-powered data cleansing, however, offers an ongoing, intelligent solution. By using machine learning algorithms trained on historical datasets, AI can detect anomalies, inconsistencies, and outliers in real-time—well before they cause issues downstream. Check out Snowflake Cortex
3. Real-Time Monitoring with Advanced Data Observability
Real-time data monitoring goes beyond simply tracking data flow; it involves using data observability tools that provide a comprehensive view of the health of your data pipelines. These tools can proactively detect issues, such as slow pipelines, unexpected data shifts, or irregularities, and trigger automated responses to fix problems before they impact operations. Imagine a dashboard that not only shows you the current state of your data, but also predicts potential issues based on historical trends and patterns. This is the power of data observability. Some great tools here are Bigeye and Elementary!
4. Implementing Data Governance for Accountability and Consistency
Data governance plays a critical role in ensuring long-term data quality. By establishing clear ownership, rules, and processes for data management, companies can maintain high standards of integrity and accountability throughout the organization. This involves defining data dictionaries, establishing clear roles and responsibilities for data stewardship, and implementing processes for data quality control and issue resolution.
The Role of AI and Automation in Ensuring Data Quality
In today’s data-driven landscape, AI and automation are not just tools—they are essential components for ensuring long-term data quality. By leveraging advanced machine learning, real-time monitoring, and self-correcting pipelines, businesses can proactively manage data quality and prevent the costly consequences of bad data.
1. AI-Powered Automation for Data Quality
AI-powered automation helps businesses move from reactive data management to proactive data quality strategies. By automating processes like data validation, anomaly detection, and data cleansing, AI models continuously scan datasets for inconsistencies and take corrective actions without human intervention. For example, machine learning models can be trained to identify and correct common data entry errors, such as typos or incorrect formatting.
2. Building Self-Healing Data Pipelines
One of the key innovations in modern data management is the development of self-healing data pipelines. These pipelines use AI to not only detect issues but also to fix certain data errors autonomously, ensuring uninterrupted data flow and quality. Imagine a data pipeline that can automatically detect and correct a schema change in a source database, preventing downstream data quality issues.
Conclusion
Bad data affects every part of your business—leading to poor decisions, wasted resources, and lost revenue. But with the right approach, these issues can be fixed. By using AI-powered data cleansing, automated pipelines, and real-time monitoring, companies can clean their data and keep it reliable. Strong data governance ensures data remains accurate as the business grows.
Investing in these solutions now helps avoid costly mistakes and sets your business up for long-term success. Don’t let bad data hold your business back.
Start building a reliable data strategy today.
Get in touch to learn how you can leverage AI and automation to ensure data quality and drive better decisions.
Transforming raw data into
actionable insights
We help businesses boost revenue, save time, and make smarter decisions with Data and AI
Sign-up now.
By clicking Sign Up you're confirming that you agree with our Terms and Conditions.