
How Is Quantitative Data About a Customer Most Accurately Characterized?
Did you know that companies that use customer data well are 23 times more likely to get new customers and 6 times more likely to keep them? Still, many businesses struggle to understand the numbers that matter the most.
Understanding quantitative data about customers isn't just about collecting numbers – it's about turning raw data into useful insights that help your business grow. Whether you're a new startup founder or an experienced marketer, understanding customer metrics can make the difference between guessing and using real data to make decisions.
Takeaways
- Quantitative customer data is measurable, fact-based, and helps show customer behavior patterns.
- Important metrics like CLV, CAC, and churn rate help you understand customer value and loyalty.
- Combining numbers with qualitative data gives you a complete view of customer experiences.
- Tools like Google Analytics and CRM systems are important for collecting and understanding customer data.
- The goal is to use data to improve customer experiences and make smart decisions.
Understanding Quantitative Data About Customers
What is Quantitative Customer Data?
Quantitative data is numerical information that measures customer behavior, preferences, and interactions with your business. This includes metrics like how often they buy, how much they spend, website visits, and satisfaction scores. Unlike descriptive or qualitative data, quantitative data gives you hard numbers that can be studied to show patterns and trends in customer behavior.
Key Characteristics of Customer Quantitative Data
Quantitative customer data is:
- Measurable and countable
- Based on facts
- Easy to analyze with numbers
- Good for tracking changes over time
- Comparable between different customer groups
Because of how structured it is, this type of data is very helpful for making data-driven decisions about customer experiences, product development, and marketing.
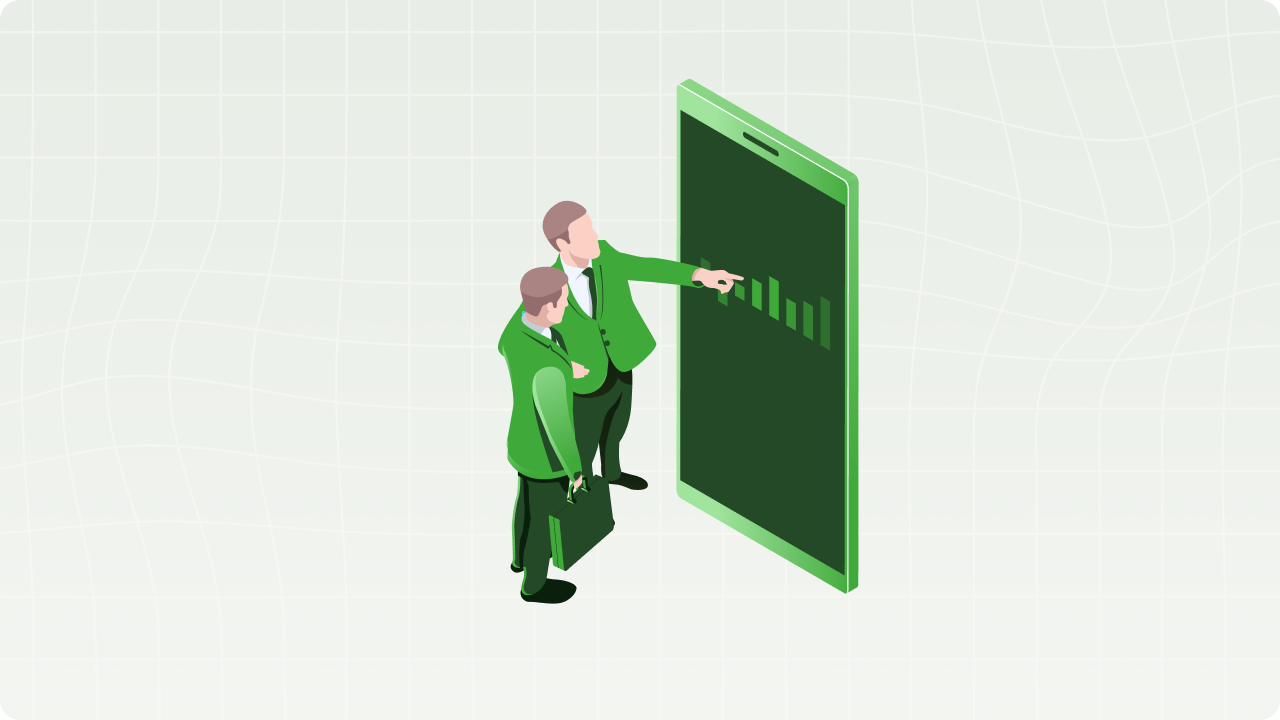
Essential Customer Metrics to Track
The most important quantitative metrics about customers include:
- Customer Lifetime Value (CLV): The total money you expect to get from a customer over time.
- Customer Acquisition Cost (CAC): The cost of getting a new customer.
- Churn Rate: The percentage of customers who stop using your product or service.
- Net Promoter Score (NPS): A measure of customer loyalty from -100 to 100.
- Customer Satisfaction Score (CSAT): A satisfaction score usually on a scale from 1 to 5.
- Average Order Value (AOV): The average amount a customer spends in each transaction.
- Purchase Frequency: How often customers make repeat purchases.
Analyzing Customer Behavior Through Numbers
When analyzing customer data, focus on:
- Purchase Patterns
- How often customers buy
- Average size of orders
- Peak shopping times
- What product categories they like
- Digital Engagement
- How much time they spend on your website
- Pages they view per session
- Click-through rates
- Bounce rates
- Customer Service Metrics
- Response times
- Resolution rates
- Number of support tickets
- Call duration
Tools for Collecting Quantitative Data
You can use different tools to gather and analyze customer data, such as:
- Analytics Platforms: Amplitude, Mixpanel
- CRM Systems: Salesforce, HubSpot
- Survey Tools: SurveyMonkey, Qualtrics
- Heat Mapping Software: Hotjar, Microsoft Clarity
- Point of Sale Systems: Square, Shopify, Amazon Seller Central
Making Data-Driven Decisions
To use customer data effectively:
- Set clear goals for measurement
- Choose the right metrics
- Establish baseline measurements
- Monitor trends regularly
- Test and validate your ideas
- Make changes based on what you learn
Combining Quantitative with Qualitative Insights
While numbers tell an important story, they work best when combined with qualitative insights, such as:
- Use quantitative data to spot trends
- Use qualitative research to understand why those trends happen
- Validate the numbers with direct customer feedback
- Build a more complete customer profile
Data Visualization and Reporting
Show customer data in easy-to-understand ways, such as:
- Interactive Dashboards: Track metrics in real time
- Trend Charts: Show how things change over time
- Heat Maps: Show how people interact with your website
- Comparative Analysis: Compare your data with industry standards
Best Practices for Data Collection
To collect high-quality data:
- Use consistent methods for measurement
- Clean and check your data regularly
- Set up proper tracking systems
- Follow data privacy laws
- Document your collection methods
- Update your metrics as your business changes
Different businesses need different approaches to data collection. The most important thing is to pick metrics that align with your business goals and track them over time to make informed decisions and improve customer experience.
Advantages and Disadvantages of Quantitative Data
Quantitative data is great for businesses because it is measurable, based on facts, and easy to analyze. It is also quick to study once collected. However, it can take a lot of time to collect large sets of data, and it might not give the deep insights you get from qualitative data, like understanding how customers feel.
Conclusion
Becoming a data-driven business starts with understanding and using quantitative customer data. By choosing and tracking the right metrics, you can find valuable insights that guide your business decisions and help you grow.
The goal is not just to collect data but to use it to improve customer experiences and boost business results. Start with a few key metrics, build a baseline, and slowly grow your data skills as you learn more.
What metrics could you start tracking today that would make the biggest impact on your business growth?
Transforming raw data into
actionable insights
We help businesses boost revenue, save time, and make smarter decisions with Data and AI