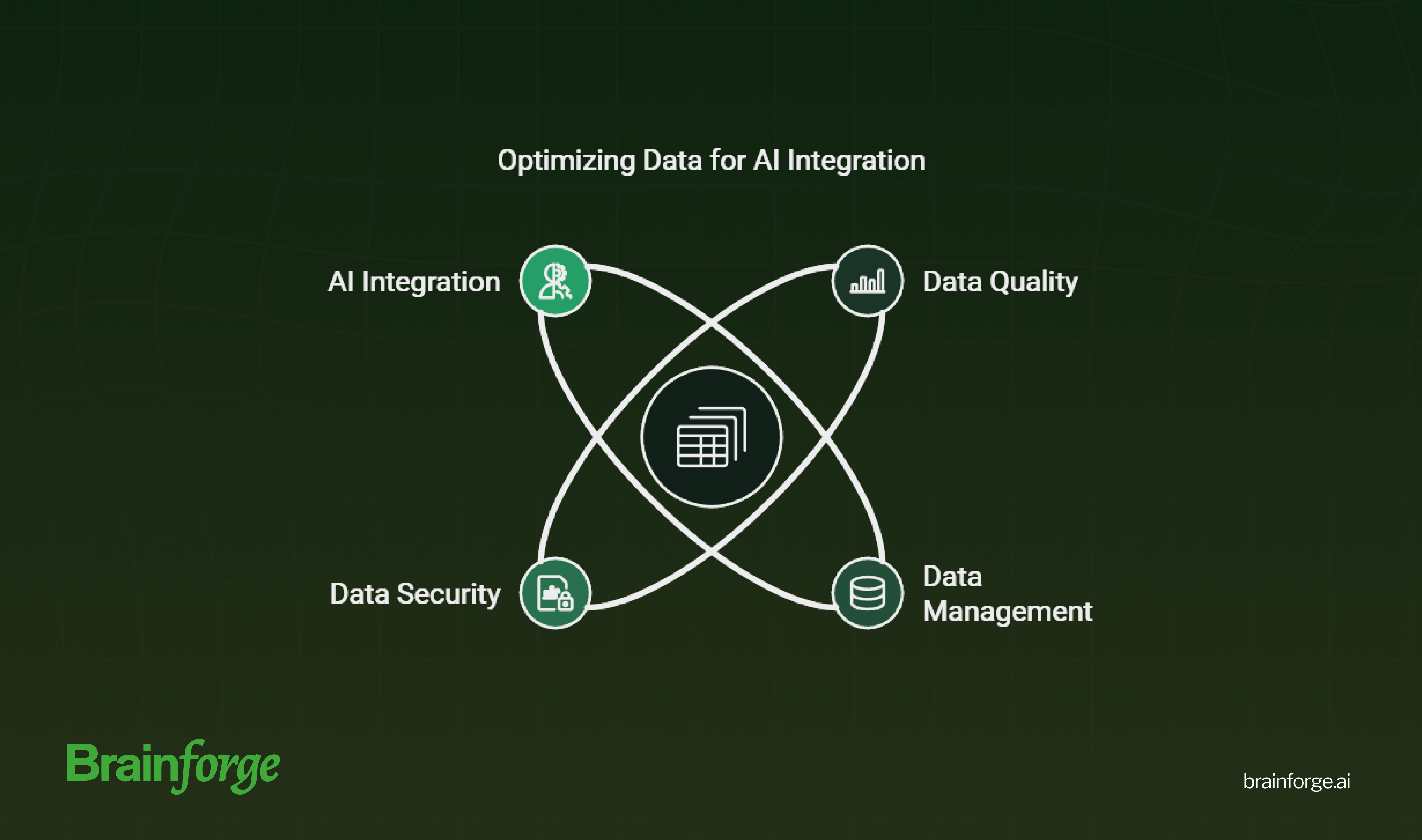
Should Companies Refine Their Data Processes Before Integrating AI?
Companies are pouring millions into AI, hoping for transformation.
But for many, that dream is turning into a nightmare.
An average of $12.4 million is lost with every failed AI project.
Think about that—a significant investment, only to watch it disappear.
Why? Surprisingly, it’s not the AI technology itself.
The problem goes deeper.
Most failures stem from poor data quality and unprepared data processes.
With 30 projected to fail by 2025, refining data isn’t optional. It’s essential for a successful strategy.
"In 2024, data quality has become the primary predictor of AI success, reducing implementation costs by 47%."–Gartner, March 2024
But what does it mean to refine data processes? And how can businesses safeguard their AI investments?
Key Takeaway
- Strengthen Data Foundations: Focusing on clean, consistent, and reliable data helps build a stronger foundation for AI.
- Boost Success Rates: Companies with refined data processes are 3 times more likely to succeed in AI projects, reducing risks, and improving ROI.
- Cut Implementation Costs: High-quality data reduces AI implementation costs by up to 50%, saving time and resources on reworking and cleaning data.
- Reduce Technical Debt: Proper data validation and quality controls prevent technical debt, making AI systems easier to maintain and more reliable in the long run.
- Build Competitive Advantage: A well-organized data ecosystem enables smarter, faster decisions and provides a competitive edge as AI becomes integral to operations.
The True Cost of Bad Data
Every AI initiative relies on data accuracy, completeness, consistency, and timeliness. MIT Sloan’s recent research reveals that unstructured data and schema inconsistencies can increase data preprocessing by 3.5 times, adding significantly to costs and time.
According to Forbes, 40% of data scientists’ time goes into cleaning data instead of building models. And IBM reports that poor data quality costs companies around $12.9 million annually.
This issue affects not only productivity; it impacts revenue, with companies losing 18% of revenue when relying on flawed data. For a $10 million business, that’s $1.8 million in lost opportunities. Stronger data governance, ETL (Extract, Transform, Load) processes, and master data management can help prevent these losses.
What, then, does a solid data foundation look like? Let’s look closer.
Why Good Data Makes or Breaks AI Success?
AI models need robust data pipelines and MLOps (Machine Learning Operations). According to Stanford’s AI Index Report, 84% of machine learning models fail because of data pipeline issues, not because of flaws in the algorithms. Structured data lakes and validation frameworks improve model accuracy, reduce data drift, and allow AI to perform reliably.
Consider predictive analytics, computer vision, and natural language processing (NLP). Each of these applications has unique data quality needs. Companies that refine their data processes before deploying AI see improved accuracy and more reliable performance.
Deloitte’s research found that strong data quality controls reduce AI-related errors by 56%, resulting in faster, more dependable systems.
Building a Strong Data Foundation
A modern data infrastructure is more than storage; it involves data ingestion, validation, and integration strategies. Microsoft reports that event-driven architectures and stream processing improve data freshness by 64%. Adding validation practices like JSON schema verification cuts data errors by 76%.
Companies with a well-organized data flow enable AI to perform at its best. McKinsey’s Digital Report shows that companies with comprehensive data frameworks experience a 3.2x increase in ROI from their AI projects.
But what happens if companies skip these steps?
The High Cost of Cutting Corners
Rushing into AI without a refined data process can have steep costs. Accenture highlights that poor data preparation leads to technical debt, often resulting in 4.3 times more time on maintenance than on model development.
Companies that refine their data processes before integrating AI find it easier to manage costs, reduce technical debt, and speed up project delivery.
But there’s one last issue to address: AI bias.
Fixing AI Bias with Better Data
AI systems exhibit bias, but improved data preparation can ease much of it. Recent studies show companies focusing on data quality report 43% fewer bias issues. This translates to a fairer, more reliable AI that earns trust and delivers results.
So, the question isn’t just about cost—it’s about building AI systems that are unbiased and trustworthy.
Conclusion
The data speaks for itself. Should companies refine their data processes before integrating AI? Yes. Successful AI projects require more than technology; they need strong, clean data. Companies that prioritize data refinement see 3 times better results and nearly 50% lower costs. The future of AI depends on the strength of your data foundation.
As AI becomes a business essential, will you choose a quick start with rough data, or a solid foundation that sets you up for long-term success?
Transforming raw data into
actionable insights
We help businesses boost revenue, save time, and make smarter decisions with Data and AI
Sign-up now.
By clicking Sign Up you're confirming that you agree with our Terms and Conditions.